Grad-PU: Arbitrary-Scale Point Cloud Upsampling via Gradient Descent with Learned Distance Functions (CVPR 2023)
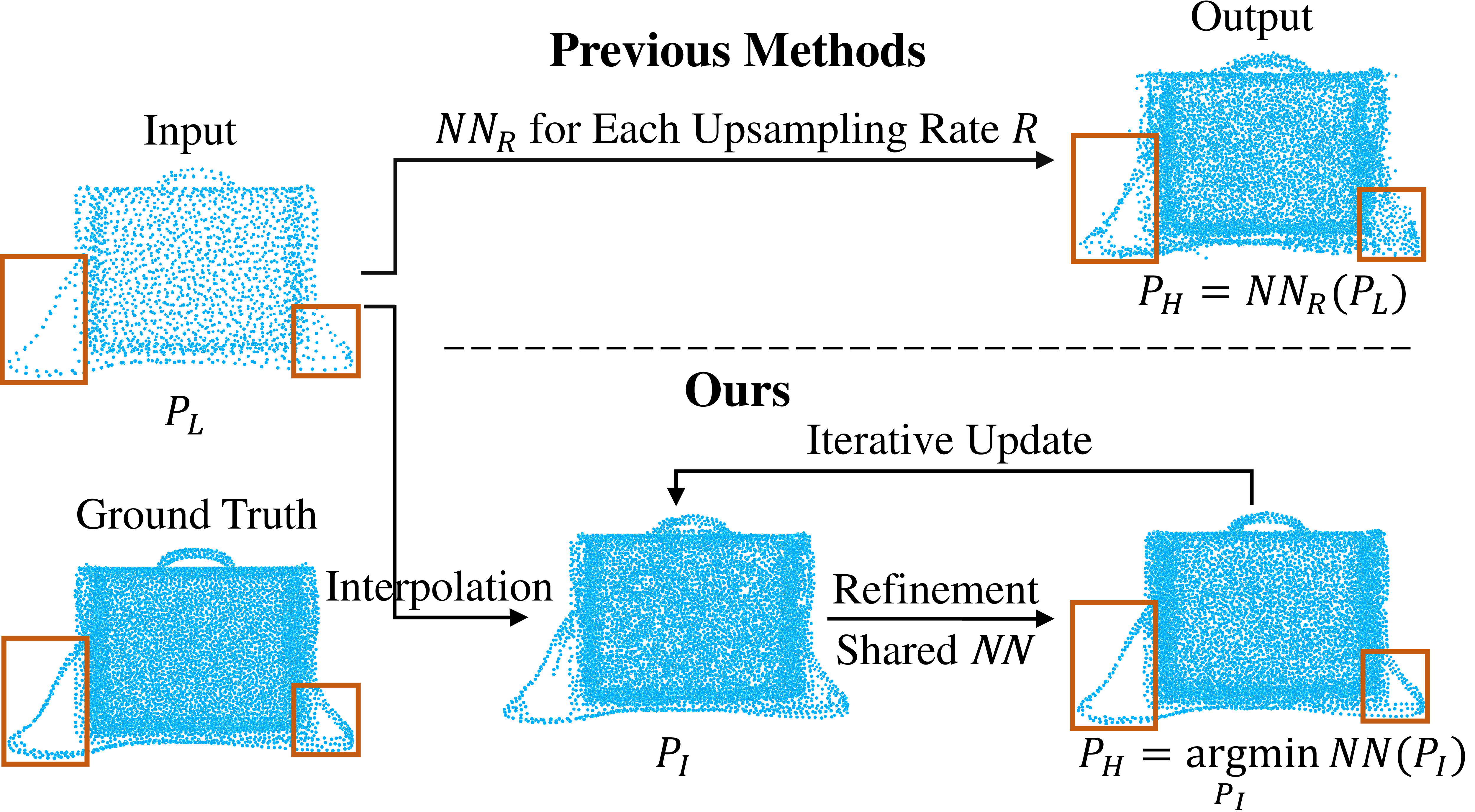
Abstract
Most existing point cloud upsampling methods have roughly three steps: feature extraction, feature expansion and 3D coordinate prediction. However, they usually suffer from two critical issues: (1) fixed upsampling rate after one-time training, since the feature expansion unit is customized for each upsampling rate; (2) outliers or shrinkage artifact caused by the difficulty of precisely predicting 3D coordinates or residuals of upsampled points. To address them, we propose a new framework for accurate point cloud upsampling that supports arbitrary upsampling rates. Our method first interpolates the low-res point cloud according to a given upsampling rate. And then refine the positions of the interpolated points with an iterative optimization process, guided by a trained model estimating the difference between the current point cloud and the high-res target. Extensive quantitative and qualitative results on benchmarks and downstream tasks demonstrate that our method achieves the state-of-the-art accuracy and efficiency.
Publication
The IEEE/CVF Computer Vision and Pattern Recognition Conference (CVPR), 2023.